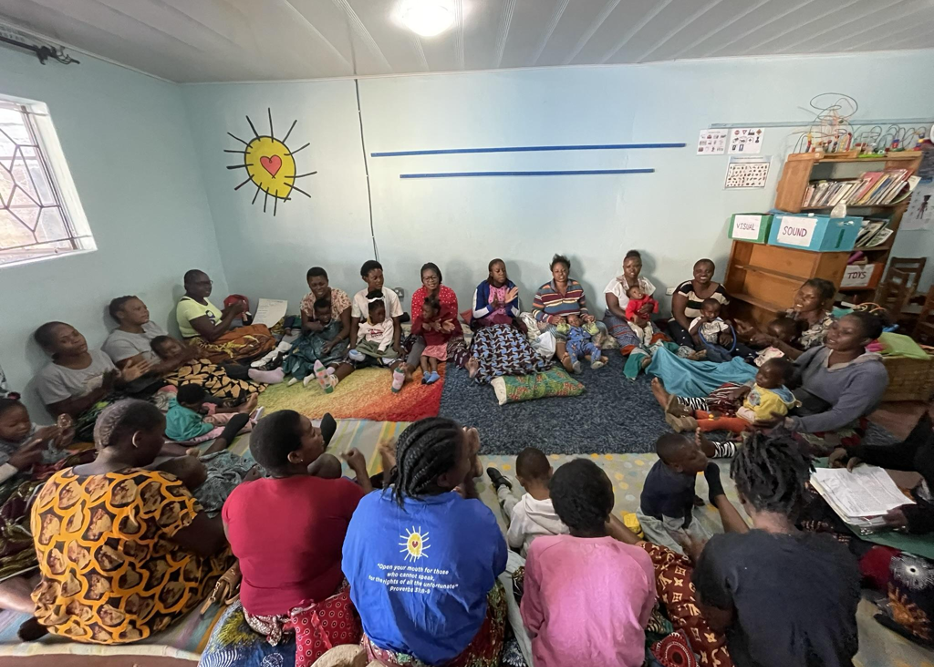
Data plays a central role in shaping policy and service provision, especially in areas such as healthcare, education, and local governance. But for many rural communities, data is collected and decisions are made without members’ input or context, leading to policies that don’t address their needs. This disparity is particularly clear when it comes to groups that are remote or facing socio-economic barriers. Having worked closely with communities through my work at Special Hope Network in Zambia, I have seen firsthand how participatory data processes can stop this disconnect, build trust, and empower communities to play an active role in shaping their future. Special Hope Network is dedicated to improving the lives of children with intellectual disabilities and their families by providing essential services, advocacy training, and community outreach. We have facilitated community-based data collection and feedback sessions, ensuring that the voices of marginalized groups are central to decision-making. This approach has not only shaped more inclusive policies but also fostered a sense of ownership among the communities we serve.
Participatory data processes can transform local decision-making by involving community members in the entire data cycle—from collection to interpretation. For example, when local health workers and residents are engaged in mapping health resources or tracking disease outbreaks, the data they generate reflects a deeper, more nuanced understanding of local challenges. These community-led efforts enhance the accuracy of the data and also build trust, as residents see their lived experiences reflected in the information that influences policies. By giving communities ownership over the data process, they feel more invested in the outcomes, leading to stronger engagement and a higher likelihood of successful implementation of policy interventions.
From data subjects to data partners
Participatory data methods are centered around involving communities actively and with clear purpose at all stages of data collection, interpretation, and use. This approach contrasts with traditional top-down methods, where external entities gather data without considering local perspectives or contexts. Examples of participatory data methods include community-led surveys, mapping exercises, focus groups, and collaborative data analysis. While these approaches may sound simple, they are often excluded from data collection processes. Yet these methods enable communities to identify issues that are most relevant to them and to use data as a tool for driving change.
This concept has had a powerful impact in Zambia, where many rural areas lack consistent representation in national datasets, skewing the development agenda towards urban needs. Through initiatives such as malaria prevention and rural health programs, I have witnessed how participatory data initiatives not only provide deeper, more contextually accurate information but also transform communities from data subjects to data partners.
For example, in Eastern Zambia, participatory data processes helped uncover malaria hotspots through local mapping efforts, where community members highlighted their experiences of seasonal infections. Instead of external observers setting the agenda, community members guided the data collection, offering a more detailed picture of infection trends than previous methods. By actively involving local voices, the data was more targeted to reflect lived realities and led to focused, season-specific malaria prevention interventions. Seeing the community directly influence the allocation of bed nets and health resources strengthened the local belief that data can be a tool for advocacy, not just observation—or worse, exploitation.
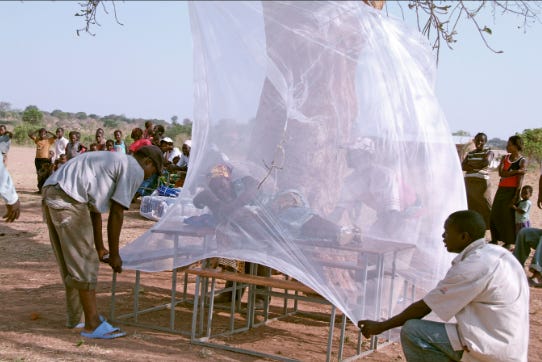
Supporting underrepresented children
In my experience with the Special Hope Network in Zambia, participatory data collection has been transformative. Caregivers and parents are engaged as respondents and collaborators, actively shaping what data is collected and how it would inform support services. We introduced a co-design process where caregivers provided input on survey design, identifying core challenges, and selecting data points. This shifted the traditional power dynamic, transforming caregivers into informed, expert stakeholders, and helped capture critical insights on barriers to accessing educational resources and understanding local attitudes toward disability.
One key insight from this approach revealed was that caregivers faced significant stigma, not just from the wider community but often within their extended families. This had not been captured in prior data, highlighting an unmet need for family-centered support. In response, we developed a new program focused on family-based education, aiming to foster inclusive attitudes starting at home.
At first, community hesitance was a major challenge, as prior one-sided data collection had bred a degree of caution. To build trust and foster transparency we knew it was important to train local facilitators from within the communities to lead data-gathering efforts and ensure community members saw familiar faces driving the process. As a result, we were able to gather more candid, and therefore more nuanced, data. Collaborative methods both enrich the quality of the data, and establish a cycle of accountability within communities.
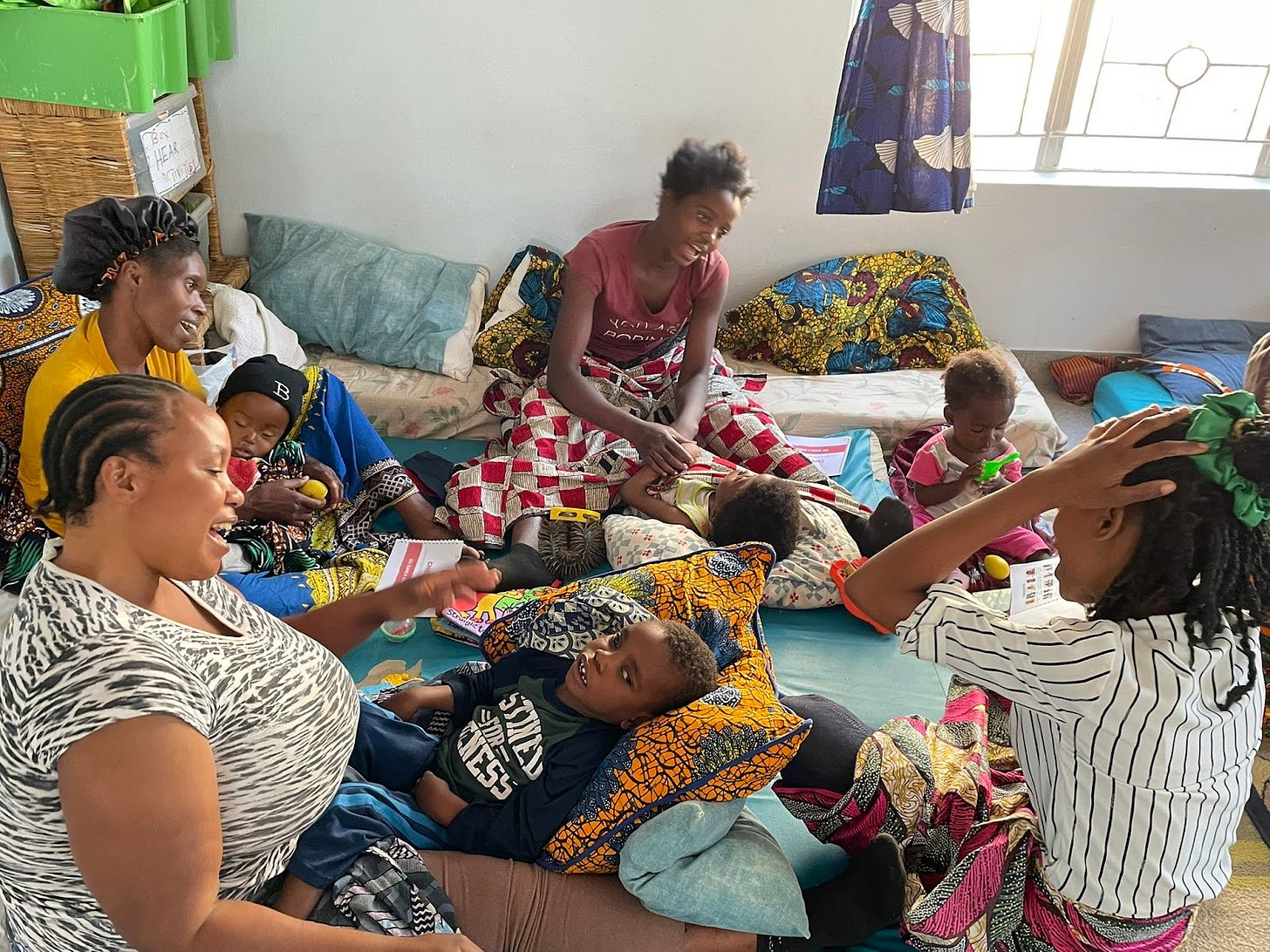
A path forward for development
Participatory data initiatives in Zambia demonstrate how communities can be empowered through inclusive, collaborative processes. For communities, rather than being relegated to the periphery, participatory data methods places them at the heart of decision-making, turning data collection into a form of advocacy and ensuring that services created are trusted, fit for purpose and effective. For development practitioners, participatory data practices are essential in promoting equity, building trust, and improving data quality, particularly in regions where communities remain underrepresented.
The challenge lies in normalizing participatory data methods as part of the development agenda. Achieving this requires structured approaches, such as training local leaders in data collection, integrating community feedback mechanisms, and establishing policies that mandate participatory practices in program design. Zambia’s experience serves as a reminder that community-driven data can be transformative, paving the way for a more inclusive data landscape. For those working in development, participatory data methods offer a way forward—helping to ensure that everyone’s needs are met and heard.